Why Molecular Docking is needed in Drug Discovery?
The discovery of a new drug is a challenging and laborious task that is further complicated by overburdening finances. Drug discovery is an extremely time-consuming process and at the same time a great risk for pharmaceutical companies. The steps involved in drug discovery are given:
Identification of a highly active target in the progression of a disease.
Identification of the candidate that can either inhibit or reverse the disease progression. In this step, the molecule is discovered which shows efficacy on a simple screen known as the “hits”. In screening, a large number of molecules are tested for a hit, so screening huge molecular libraries are to be maintained, shooting up the cost. These compounds are referred to as “leads”.
Synthesis of the compound with minimal toxicity, high bioavailability, and cost-effectiveness.
Finally, production for distribution in the market.
From the perspective of an academician, this method is highly costly especially time and cost needed for high throughput screening (HTS), thus, making it not feasible. Also, from an industrial point, it has limitations associated with high costs and failures. The true drivers of pharmaceutical industries are innovative drugs but the past decade has seen a small amount of new molecular entities (NMEs).
The challenges faced in conventional drug discovery are curbed through molecular docking, an exemplary tool. In molecular docking (MD), to find hits from existing chemicals, Virtual screening (VS) is done. This viable alternative to HTS, not only reduces the cost but also can screen thousands and millions of compounds in just a few days. In addition to this, VS also allows initiating projects for newer targets for which no data is previously available. Thus, molecular docking is a resource-saving technique for, both academia and industries, novel drug discovery and development.
But before going into detail about molecular docking, it is important to mention some of the chemical databases that play an essential role in computer-aided drug discovery. These chemical databases provide us with information that can be used for screening for drug design and discovery. Major chemical databases are given by following table 1:
Table 1: Major Chemical Databases
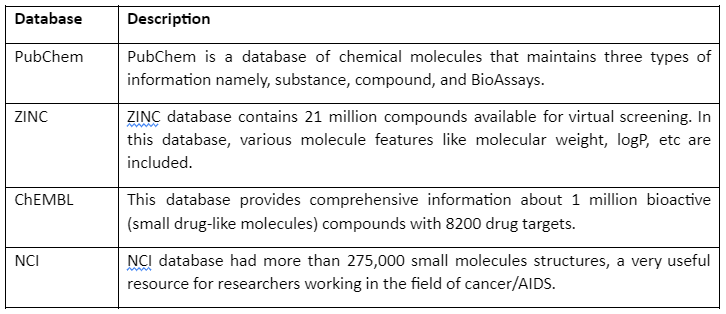
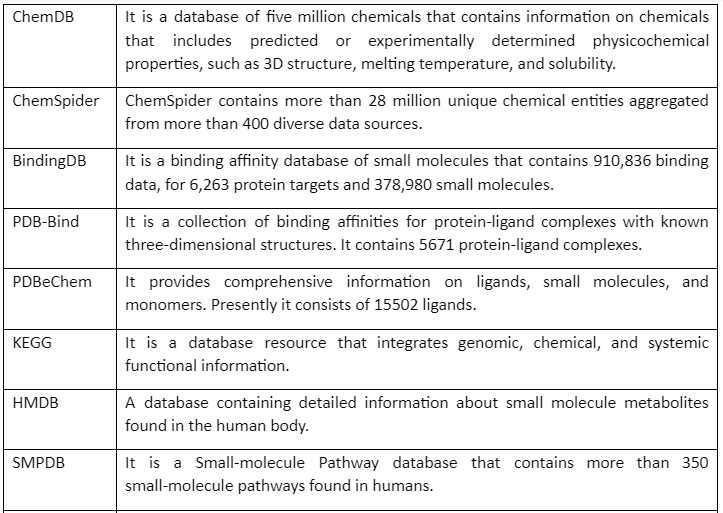
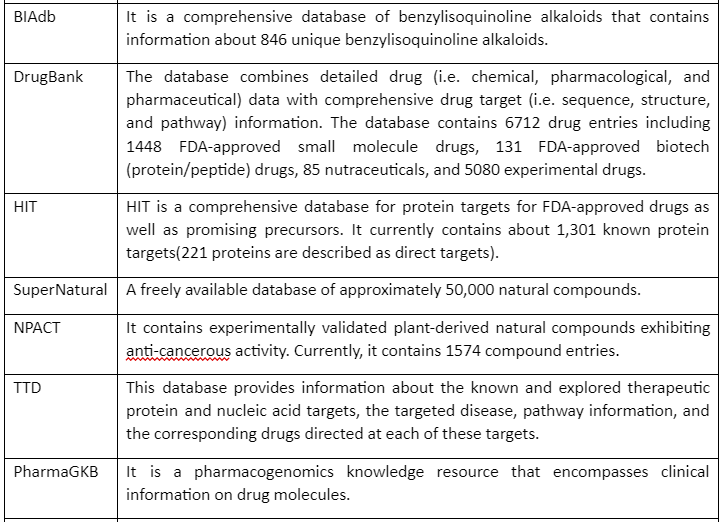

Molecular Docking for Drug Discovery
Any two types of molecules can interact with each other in multiple ways, not only the interaction of the protein with protein but also protein with small molecules. MD plays a crucial role in the prediction of the intermolecular framework formed between two proteins or between a protein and a small molecule. MD, then suggests many binding modes through which the protein can be inhibited. The docking study required a high-resolution X-ray, a homology-modeled structure that has predicted or known binding sites in the biomolecule. Docking methods find out the correct hit by fitting ligand in the binding sites by combining and optimizing many variables like hydrophobic, steric, and electrostatic complementarity. It also estimates the free binding energy which is known as scoring.
Types of Docking
Molecular docking is of the following types:
Rigid ligand and rigid receptor docking
In this type of docking, ligand and receptor molecules are treated as rigid bodies and hence the space of search is quite limited i.e., only three rotational and translational degrees of freedom. In such cases, the flexibility of the ligand molecule is sorted by using a set of pre-computed ligand conformations or by enabling a degree of atom-atom overlap between protein and ligand. The earlier versions of software like DOCK, FLOG and some of the protein-protein docking programs like FTDOCK make use of this method for molecular docking.
Flexible ligand and rigid receptor docking
In this type of molecular docking, the ligand and receptor are considered flexible and rigid entities, respectively. This is based on the assumption that to bind, ligand and receptor molecules change their conformations for making a complex at a minimum energy cost. The cases where the receptor is also considered rigid, the energy cost will become quite high. This approach saves computational time and carries out docking at high accuracy. Almost all the tools of docking have adopted this method like FlexX, Autodock, etc.
Flexible ligand and flexible receptor docking
This third of docking considers both ligand and receptor molecules as flexible entities. As per Teague’s review, the intrinsic ability of proteins to mobilize is associated with the behavior of ligand binding. Incorporating the flexibility of ligand is quite challenging due to energy calculations and taking into account all the degrees of freedom at a suitable computational time. Molecular docking can model all the degrees of freedom in receptor and ligand into a complex but the computational expense is quite high which makes this method less popular and used.
There are many methods available that implement receptor flexibility. Some of them are given in table 1:
Table 1: general methods for implementation of receptor flexibility
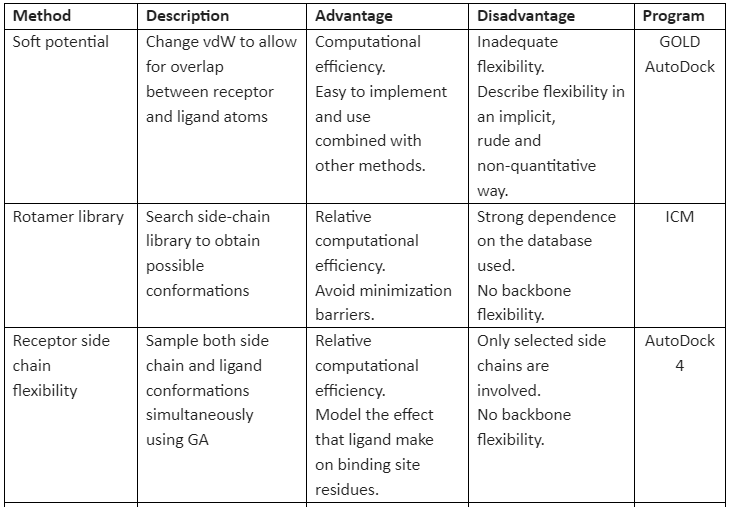
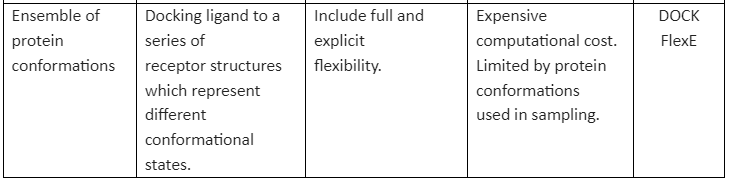
Algorithms and Functions
To do molecular docking, many software is available. The software is distinguished based on the type of algorithm and scoring function. The description of different algorithms and scoring functions is given below:
Sampling algorithm
As mentioned earlier, the binding between two molecules has a high number of possibilities, and although, there are advanced computing technologies it would be very time-consuming and expensive to produce all possible modes of binding. To concise, the number of options, different types of algorithms are designed.
Several algorithms are present and they can be categorized according to the degree of freedom they do not consider. Some of them are:
Matching Algorithm (MA):
The simplest algorithm developed considered molecules as two firm or rigid bodies, this considerably reduced the degree of freedom to just six cases i.e., three translational and three rotational. The well-known program that employs this algorithm is DOCK. The DOCK was developed to find molecules that have a high similarity of shape to the binding sites pockets/grooves. It works by deriving the snap of the predicted /anticipated binding site that is present on the surface of the protein. This snap/image has many overlapping spheres of variable radii which touch the macromolecule molecular surface at only two points. The ligand molecule is also thought of as a set of spheres that can almost fill the space occupied by the ligand. After the representation of both protein surface and ligand as the sphere is done, then the pairing rule is applied. The principle of the pairing rule is that the ligand sphere can be paired with the protein sphere if the internal distance of all the spheres in the ligand is set to match all the internal distances in protein ser, allowing some user-specific tolerance. Thus, in this way geometrically same sphere clusters are identified on the protein site and ligand molecule. The MA was used by other software like Ph4DOCK, LibDock, SANDOCK, LIDAEUS, etc.
MA has advantages like high speed and efficiency but there are many limitations. One of them is the need for receptor geometry before docking and also due to the absence of molecular flexibility, it is unable to explain ligand-protein interaction properly.
Increment Construction:
In this algorithm, the ligand is fragmented from rotatable bonds into many parts/segments. The receptor surface will be anchored by one of the segments. This anchor is mostly considered as the fragment which shows a maximum number of interactions with the receptor surface and also it has minimum alternate confirmations making it quite rigid like the ring system. After the establishment of the anchor, e of the fragment is added one by one. Most of the time those fragments are added on a priority basis which has higher chances of depicting interactions like hydrogen bonding as their nature is directional and they determine the specificity of ligand. Also, the H-bonding makes an accurate prediction of geometry. After the particular fragment is added, the positions with small energies are considered for the next iteration, resulting in a robust and fast algorithm. The software that employs IC is SLIDE, FlexX, DOCK 4.0, eHiTS, Hammerhead, PatchDock, SKELGEN, FLOG, ProPose, etc. The main limitation of IC is its restricted medium size ligands and it is not suitable for large size ligands.
Monto Carlo (MC):
This is a very useful algorithm. In MC approach, the ligand is changed or modified slowly by using the rotation of the bond and translation or rotation of the full ligand. For modification, we can change more than one parameter at a time to get the required confirmation. After that, conformation is tested at the binding site. Then, it is accepted or rejected for the next iteration based on Boltzmann’s probability constant through the calculation of energy by using molecular mechanics. The acceptance or rejection of a ligand with specific conformation is a function of change in energy w.r.t. a parameter “T”. this parameter is interoperated as temperature (simulated annealing). This method is unique from the other approaches due to this principle of acceptance or rejection. In addition, to this, the MC algorithm favors an increase in energy as compared to other algorithms which favor a decrease in energy. MC approach has a fascinating spin-off known as the Tabu search; its main purpose is to maintain the search space record of the binding site which has been already done. This Tabu search allows the maximum exploration of the binding site in addition to saving time. The programs that incorporate MC are GlamDock, DockVision 1.0.3, QXP, PRODOCK, ICM, Yucca, RiboDock, ROSETTALIGAND, AutoDock FDS, etc. The main limitation of MC is the convergence uncertainty. This can be improved by performing numerous independent runs.
Genetic Algorithm (GA):
This algorithm is very similar to the MC approach. This method is mostly used to find out the global minima and it is inspired by Darwin’s theory of evolution. GA keeps a ligand population that has a linked fitness determined by the scoring function. Each of the ligand molecules depicts a potential hit. The GA modifies the ligands of the population by either crossover or mutation. In stage one of GA, a new population is generated by accessing, and then the more fit ligands are selected from the prior population. The population members are then transformed in the modification step. The mutation operator will then produce new ligands from a single ligand by changing fragment representation randomly. On the other hand, the crossover operator exchanges information among the two population members. The GA approach has been employed in Autodock 4.0, FITTED, FLIPDock, GAsDock, PSI-DOCK, GOLD 3.1, DARWIN, DIVALI, GAMBLER, etc. The limitation of GA is also similar to MC where the convergence uncertainty is the problem.
Hierarchical method:
In this approach, the conformation of the ligands having low energy is pre-computed and aligned. The populations of the pre-generated ligand conformations are combined into a hierarchy such that the same conformations are placed next to each other in the hierarchy. After that, when the translation or rotation of the ligand is carried out, the docking program will use this hierarchical data structure and curtail the potential outcomes. For example, take an atom that is present near the ligand and the rigid center of the ligand antagonizes as the protein is rotated or translated, this approach will not only reject this ligand but also all of them present in this specific hierarchy for that specific conformation under observation. The software that uses this method is GLIDE.
Scoring functions
The algorithms are supplemented by scoring functions. In VS, the assessment and ranking of predicted conformation of the ligand is a very important aspect. In cases where our interest is only to understand how a single ligand molecule binds with a biomolecule, then in this case scoring functions will enable us to predict docked orientation. It will show the intermolecular complex’s “true” structure with accuracy. On the other hand, if we want to assess multiple ligands, in this case, the scoring function should not only recognize the “true” docking pose but also it should be able to rank ligands relative to each other. Therefore, scoring functions are essential for ranking different poses.
The scoring functions make use of many assumptions and simplifications to predict the binding energy of the complex in a short time. The most widely used scoring function keeps a balance between the accurate prediction of binding energy and computation cost measured by time. Many scoring functions have been developed in past years but they can be categorized into three main groups:
Force field functions (FF):
As the name indicates, FF scoring functions are based on physical atomic interactions like electrostatic interactions, van der Waals interactions and bond angles, bond lengths, and torsions. the programs which have incorporated the FF scoring function are GoldScore, ICM SF, DockScore, QXP SF, HADDOCK Score, etc.
Empirical scoring functions:
This scoring function is based on the assumption that the binding energies of a complex can be estimated by a sum of individual uncorrelated terms. The calculation of binding energy is done using coefficients of many terms which in turn are obtained from regression analysis using experimentally found binding energy or potentially from x-ray structure information. The terms in empirical scoring functions are simpler as compared to FF scoring functions, therefore they can do binding score calculations at a much faster pace. By using many diverse empirical energy terms, many different types of empirical scoring functions are developed like RankScore, ChemScore, SCORE2, LigScore, HINT, etc. In the empirical scoring function, all the diverse energy terms are taken into account due to which the chances of double-counting the same energy term arises and it is very difficult to handle.
Empirical scoring functions:
This scoring function is based on the assumption that the binding energies of a complex can be estimated by a sum of individual uncorrelated terms. The calculation of binding energy is done using coefficients of many terms which in turn are obtained from regression analysis using experimentally found binding energy or potentially from x-ray structure information. The terms in empirical scoring functions are simpler as compared to FF scoring functions, therefore they can do binding score calculations at a much faster pace. By using many diverse empirical energy terms, many different types of empirical scoring functions are developed like RankScore, ChemScore, SCORE2, LigScore, HINT, etc. In the empirical scoring function, all the diverse energy terms are taken into account due to which the chances of double-counting the same energy term arises and it is very difficult to handle.
Knowledge-based scoring functions:
The basis of the knowledge-based scoring functions is that they are derived from the structural information which is present in the experimentally determined atomic structures. This scoring function uses statistical analysis of complexes’ crystal structure to get interatomic contact frequencies between ligand and protein molecule founded on the belief that the stronger the interaction between the two, the greater is the frequency of occurrence. The widely used knowledge-based function are DrugScore, SMoG, ITScore/SE, MScore, BLEEP, etc. the major advantage of this function is the computational simplicity in the case where the database is large. On the other hand, the accuracy of reference state prediction and under-representation of metals and halogens is the main limitation.
The molecular docking in drug discovery is illustrated below:
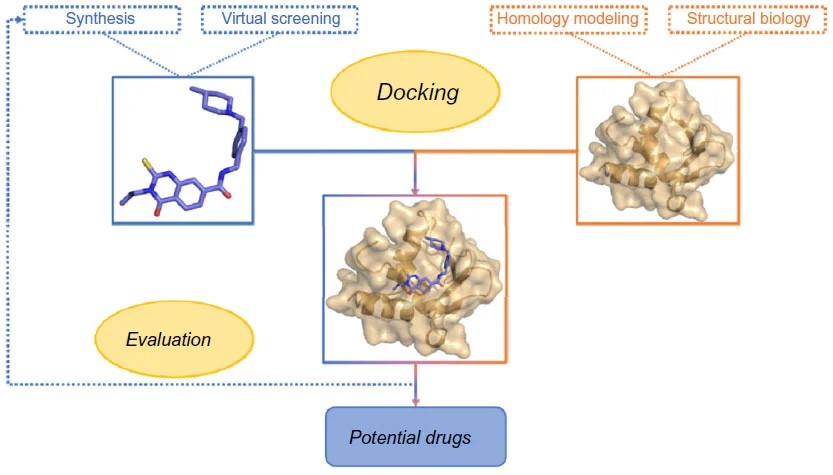
Overview of socking in drug discovery
Molecular Docking and developed Drugs
Some of the drugs developed through docking are listed below:
HIV 1 Integrase—A new drug treating AIDS, discovered by Schames and coworkers.
α1A Adrenergic receptor—Evers and colleagues generated a model of the receptor.
Type I TGF-beta receptor kinase— Novel Type I TGF-beta receptor kinase inhibitor.
Checkpoint Kinase 1—Lyne and colleagues discovered Checkpoint Kinase 1 (Chk-1) inhibitors.
Human aldose reductase (ALR2)—ALR2 catalyzes
Limitations
The major limitation of molecular docking is:
Lack of confidence in scoring functions to give accurate binding energies.
Unsolved problem of a water molecule in the binding pocket during docking.
Rigid receptor considerations.
Off-target effects.
Conclusion
Many novel drugs have been synthesized by using molecular docking, as some of the examples are quoted above. It is without any doubt, an efficient, cost-effective, and time-saving approach to drug discovery. But still, many challenges are present which need to be addressed and improved.